Qualitative data analysis is the work of organizing and interpreting descriptive data. Interview recordings, open-ended survey responses, and focus group observations all yield descriptive—qualitative—information. This is the opposite of quantitative data, which is all about numbers and statistics.
Qualitative data can’t easily be cleaned, sliced, and diced like its numerical sister. So researchers use specific qualitative data analysis methods to understand the information they collect.
The field of research recognizes five qualitative data analysis methods. We’ll quickly define each one. Then we’ll break down how to use them, when, and why.
#1. Content Analysis
Content analysis is when researchers categorize and organize words, concepts, patterns, and themes in their data.
When You Should Use Content Analysis
Content analysis is useful for identifying trends and patterns in research.
The trends could be literally anything. Market researchers could look at a large sample of contemporary ad campaigns to spot trends in how businesses use emotions to appeal to their customers.
Social researchers could study mommy blog posts and Instagram momfluencers from 2010 to 2020 to identify patterns about how motherhood changed in the span of a decade.
You get the idea.
Content analysis hasis flexible, and to better understand it, get to know the two subtypes of content analysis: conceptual analysis and relational analysis.
First, let’s look at conceptual analysis.
When people talk about content analysis, they often mean conceptual analysis. This method involves picking a concept and then counting how often it appears in your data. The basic goal is to see how frequently certain terms come up. In general, conceptual analysis requires you to do three things:
- Identify your question: Figure out what you want to learn from your research and choose your samples accordingly.
- Determine your level of analysis: Decide whether you want to analyze words, phrases, sentences, or themes.
- Code the text: Break the text down into manageable categories. In other words, figure out which specific words or patterns are relevant to your research question, and quantify them. This process, called coding, is made much easier with qualitative research software like QDA Miner and ATLAS.ti.
Relational analysis takes conceptual analysis a step further. It involves identifying patterns and focusing on the relationships between them, rather than studying the existence of the patterns themselves.
There are three subtypes of relational analysis:
- Affect extraction: Identifying and analyzing the emotions expressed within your qualitative data.
- Proximity analysis: Looking at how often and where words, phrases, or ideas appear close to each other in a text.
- Cognitive mapping: Using charts, graphs, or other visualization tools to explore the relationships between specific themes and trends.
When To Use Content Analysis
How do you know when to use cognitive vs. relational concept analysis?
It’s simple.
- Use conceptual analysis when you want to quantify the presence and frequency of a specific concept.
- Use relational analysis when you want to explore the relationships between concepts in a set of qualitative data.
Say you run a survey to ask a group of dog owners whether they let their furry friends sleep in their beds with them. Conceptual content analysis can reveal how many times certain words are used, like “always,” “never,” “cuddle,” and “scratch.” It can also show you trends in the attitudes pup parents have about bedsharing (or not) with their furbabies.
Relational analysis can help you explore themes among people who responded “yes” and people who said “no.” This, in turn, can help you understand the reasons behind the answers.
#2. Narrative Analysis
Narrative analysis involves collecting stories or accounts and looking for underlying themes.
When You Should Use Narrative Analysis
Use narrative analysis when you need to understand the stories behind client or customer experiences.
If your goal is to improve your customer journey, for instance, narrative analysis can reveal what your customers think about the journey as it is right now. What they love about it—and what they don’t.
The content you need for narrative analysis might already be out there. Do your customers or clients give reviews? Those count as narratives that can be analyzed.
But you can also be intentional and methodical about collecting the qualitative data you need for these reviews. Consider hosting one-on-one interviews with a small group of customers. Or running a focus group. Even surveys with space for long-form responses provide narratives you can dig through.
An Example of Narrative Analysis
Imagine a mid-sized retail company, Fashion ABC, is facing a decline in repeat customers. This is despite offering high-quality products—and even getting top influencers to be brand ambassadors. For some reason, though, Fashion ABC just can’t hang onto as many customers as they’d like to.
To get to the bottom of this, they decide to use narrative analysis on customer feedback collected over the past year.
Here’s how Fashion ABC runs the process, step by step.
- Gather stories: Fashion ABC collects customer stories from reviews, social media comments, and customer service call transcripts.
- Find the narratives: The team looks for personal stories within the data. They note the positive stories but focus more intensely on any narrative with a negative emotion, like anger or frustration.
- Identify themes: Next, Fashion ABC categorizes these stories to spot common themes, like “return process,” “customer service,” “product quality,” and “shopping experience.”
- Analyze patterns: In the “return process” category, the Fashion ABC team notices a trend: many customers are unhappy with the confusing return policies and especially the slow refunds.
- Draw insights: It becomes clear that although customers love the products, the complicated return process is a major pain point. Even returning something as simple as a too-big shirt or pair of pants is a major hassle. This issue shows up consistently across different feedback sources.
- Take action: Fashion ABC decides to simplify their return policy. They make it clearer and look to other companies, like Target and Costco, to figure out how to quickly refund customers. They roll out the new, easy-to-understand return policy on their website and streamline the return process.
- Check results: The narrative analysis isn’t done yet. After a few months, the Fashion ABC team gathers new customer feedback. Their goal? To see if the changes have made a positive impact on customer satisfaction and loyalty.
If the changes work, the team knows that the inefficient return process really was the culprit. If not, they can go back to square one and keep digging.
#3. Discourse Analysis
Discourse analysis looks at how people structure and express language within a cultural context.
When You Should Use Discourse Analysis
Discourse analysis is a great tool to use when you want to understand how language shapes and reflects us. It’s ideal for examining how different groups or institutions communicate—and helps measure the impact of language choices.
Take political speeches, for example. Politicians hire speechwriters for a reason. They’re looking for people who can carefully use words to influence public opinion and persuade people to a specific point of view. (Hot tip: If you’ve never done discourse analysis before, these speeches are a great place to do some practice analysis.)
Like any type of concept analysis, though, discourse analysis is helpful in just about every field.
In education, it can highlight how the language teachers use affects learning. In healthcare, it can help researchers understand how language between doctors and patients impacts the quality of care.
It’s also really useful in media studies. You can use it to look at how news outlets frame specific stories, which can reveal hidden biases. All it takes is looking at one news story from the point of view of five different publications to see how the language used in each one might influence the reader to a specific viewpoint.
In short, discourse analysis doesn’t just help you understand what is being said. It also helps you understand why it’s being said the way it is.
An Example of Discourse Analysis
Let’s pretend we’re analyzing an ad for a new herbal tea. Here’s the general process we’d take for discourse analysis.
First, we’d choose a specific ad promoting a brand of herbal tea.
Then we’d look for recurring themes within the images. Does the ad emphasize relaxation? Health benefits? Luxury? All three?
Let’s look at the image below, which shows a billboard for Pukka tea. Specifically, for a tea that’s meant to help people get ready for a good night’s sleep.
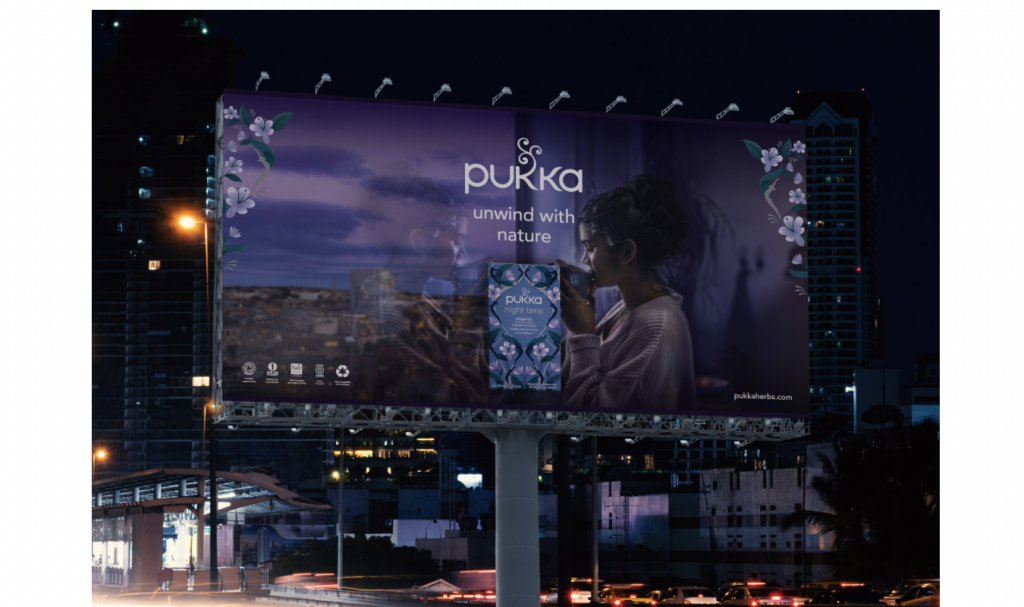
Let’s look at what words and phrases the company uses to communicate these themes.
There’s not a lot on the billboard, but what’s there is powerful: “Unwind with Nature.” Pukka’s signature twisty vines and flowers bookend the corners of the billboard, and the purple hues and steam rising from the cup help complete the ad.
The language here is telling us that with Pukka tea, we can relax and look forward to a good night’s sleep. It also suggests that by drinking Pukka tea, we’re connected to nature—even in the middle of a city.
#4. Thematic Analysis
Thematic analysis involves looking at a set of qualitative data, like interview transcripts or survey responses, and extracting meanings and themes from it.
When To Use Thematic Analysis
Thematic analysis is helpful for finding ideas—aka themes—in qualitative data. You can use it to analyze things like interview transcripts, open-ended survey responses, focus group discussions, and just about any type of qualitative data you collect or source from elsewhere.
And with today’s AI-powered tools like NVivo and ATLAS.ti, thematic analysis is easier than ever. (More on how to use AI for content analysis in a moment.)
Thematic analysis is especially useful early on in your research, especially when you’re trying to generate new ideas. Or when you’re exploring a topic without any specific hypotheses in mind.
For example, if you’re in healthcare research, you might use thematic analysis to understand how patients feel about a new treatment. In education, it can help you explore what teachers think about a new curriculum. In the business world, it’s useful for analyzing customer feedback to spot common complaints.
An Example of Thematic Analysis
Let’s say we’ve decided to use thematic analysis to analyze customer feedback for our new coffee shop. To begin, we collect and read through 50 customer reviews. Our goal is to look for recurring words or phrases that point to specific ideas—things like “friendly staff,” “cozy atmosphere,” and “long wait times.”
Next, we’ll group these elements into broader themes. “Friendly staff” and “cozy atmosphere” go in a “Positive Experiences” category. Points like “pricey menu” and “long wait times” go under a “Needs Work” category.
From there, we can summarize our findings and use them to make improvements to our new coffee shop.
#5. Grounded Theory
Grounded theory is a way of trying to understand the meanings of peoples’ actions—based on their own interpretations of those actions.
When You Should Use Grounded Theory
You should use grounded theory when you’re trying to use your data to develop a theory, rather than the other way around. Grounded theory is particularly useful when existing theories about something don’t really fit, and you’re looking for a different angle or answer.
For instance, let’s say you’re studying how people adapt to remote work in a field like telehealth. With grounded theory, you can use participants’ actual experiences and interactions to generate theories and better understand the topic of your research.
So why is it called grounded theory, anyway?
The answer lies in this definition of grounded theory, which comes to us from a 2021 article titled, “Grounded theory: what makes a grounded theory study?” in the European Journal of Cardiovascular Nursing: “The focus of [grounded theory] is to generate theory that is grounded in data and shaped by the views of participants.”
In other words, it’s a qualitative research method that invites theory to originate from the ground up, instead of the other way around.
An Example of Grounded Theory
Imagine you want to develop software to help digital nomads keep track of their working hours and block distractions. (For reference, digital nomads are people who work remotely using technology and travel as they work.)
There’s not much existing research on how these folks manage their work-life balance or connect with other people. It must be hard, since they’re always on the go. How do they do it? What does it mean for their work-life balance? You want to use grounded theory to learn more about this growing segment of people so your product can support their needs.
To collect data, you start by surveying a variety of self-proclaimed digital nomads. You also spend time observing online forums like Reddit to get more of an insider perspective.
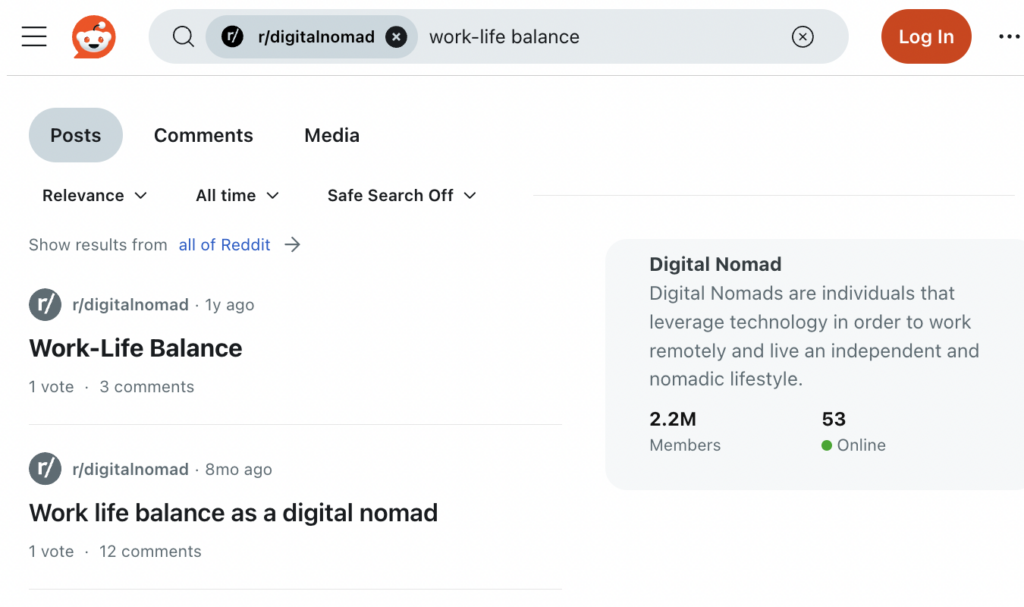
As you dig into the survey responses and your observation notes, you start the coding process. To do this, you identify concepts like “flexible work hours,” “isolation,” “community support,” and “travel logistics.”
Next, you connect these concepts into broader categories. For instance, “flexible work hours” and “travel logistics” might merge into a category called “lifestyle management,” while “isolation” and “community support” could come together under “social dynamics.”
Finally, you refine these categories into a new, core theory. You might come up with the idea that digital nomads thrive by balancing autonomy and flexible scheduling with community support. This theory highlights how digital nomads create routines that help them manage work and travel. At the same time, they rely on online and physical communities to fill their social cup.
Your grounded theory suggests that the sustainability of the digital nomad lifestyle hinges on balancing personal freedom, structured but flexible work hours, and social connectedness.
At the end of this journey, you’ve decided to build software that provides digital nomads with a one-stop shop for logging work hours, blocking distractions, and connecting with other nomads.
How to Use AI With Qualitative Data Analysis
AI is a hot-button topic, but there’s no question it can help with qualitative analysis. It can’t—and shouldn’t—do all the work for you, though.
Here’s a quick round-up of Do’s and Don’ts when it comes to using AI in qualitative analysis.
Do:
- Use AI for data cleaning: AI tools can do the mindless tasks humans take forever to do, like transcribing interviews, removing duplicates, and organizing qualitative data.
- Use AI for initial coding: AI can also do a great job helping you with the coding process. How? By identifying frequent terms in the text. Some tools can help you organize those terms into broader theme groups, too.
- Use AI for visualizing data: AI can help you create graphs and other visual representations of data, which makes it easier to digest and study.
Don’t:
- Use AI for anything else. No, really. AI is not human, and it can’t make interpretations, draw conclusions, or basically do any of the heavy thinking necessary in qualitative analysis. It’s great for eliminating busywork, but you’ve got to use your own brain and experience as a human to do all the rest.
Tools like ATLAS.ti, Nvivo, and Tableau can help you with the AI-friendly parts of qualitative research. Trust your experience as a human being to help you with the rest.